Top considerations for addressing risks in the OWASP Top 10 for LLMs
Welcome to our cheat sheet covering the OWASP Top 10 for LLMs. If you haven’t heard of the OWASP Top 10 before, it’s probably most well known for its web application security edition. The OWASP Top 10 is a widely recognized and influential document published by OWASP focused on improving the security of software and web applications.
Download the Full Report Here.
An LLM is a type of AI model designed to understand and generate human language. LLMs are a subset of the broader field of natural language processing (NLP), which focuses on enabling computers to understand, interpret, and generate human language. What makes LLMs interesting to development teams is when they’re leveraged to design and build applications. And what makes them interesting to security teams is the range of risks and attack vectors that come with that association! We’ll look at what OWASP considers the top 10 highest risk issues that applications face using this new technology.
Confidential Access to AI models and data with SafeLiShare: A Critical Necessity
In today’s digital landscape, ensuring confidential access to sensitive data is paramount. SafeLiShare offers a robust solution, extending the standard Public Key Infrastructure (PKI) without compromise. Leveraging Secure Enclave technology in the cloud, also known as confidential computing, SafeLiShare provides a secure environment supported by major cloud hardware providers such as Intel, AMD, and Nvidia. Instances running on AWS, Azure or GCP platforms deliver the highest level of security, equivalent to FIPS140-2 Level 4 standards.
End-to-end encryption, particularly encryption in use, plays a pivotal role in mitigating risks associated with OWASP vulnerabilities in Language Model Models (LLMs) and AI computation. By encrypting data before sharing and maintaining a chain of custody, SafeLiShare ensures security from the core, thwarting potential breaches.
Traditional cloud security measures, while employing a layered approach, often fall short in preventing breaches and identifying the root cause. Solutions like Cloud Native Application Protection (CNAPP) or Cloud Workload Protection Platform (CWPP) are too complicated to manage and lack the agility required in dynamic environments. It’s time to explore ConfidentialAI technology from SafeLiShare to overcome the reported vulnerabilities in LLMs.
Exploring LLMs and RAG
An LLM, or Language Model Model, is an AI model specifically designed to comprehend and generate human language. LLMs operate within the broader realm of Natural Language Processing (NLP), enabling computers to interpret, understand, and generate human language effectively. Development teams leverage LLMs to design and build applications with enhanced linguistic capabilities. However, this association introduces various risks and attack vectors, making LLMs a point of interest for security teams. RAG, or Retrieval-Augmented Generation, pertains to AI models’ capability to retrieve and generate data, enhancing their contextual understanding and generating more accurate responses.
In conclusion, safeguarding data integrity and confidentiality is paramount in the realm of AI development and data sharing. SafeLiShare’s ConfidentialAI platform offers a robust solution to mitigate risks associated with LLMs and AI computation, ensuring secure access control and data privacy.
To schedule a demo, visit SafeLiShare.
Suggested for you
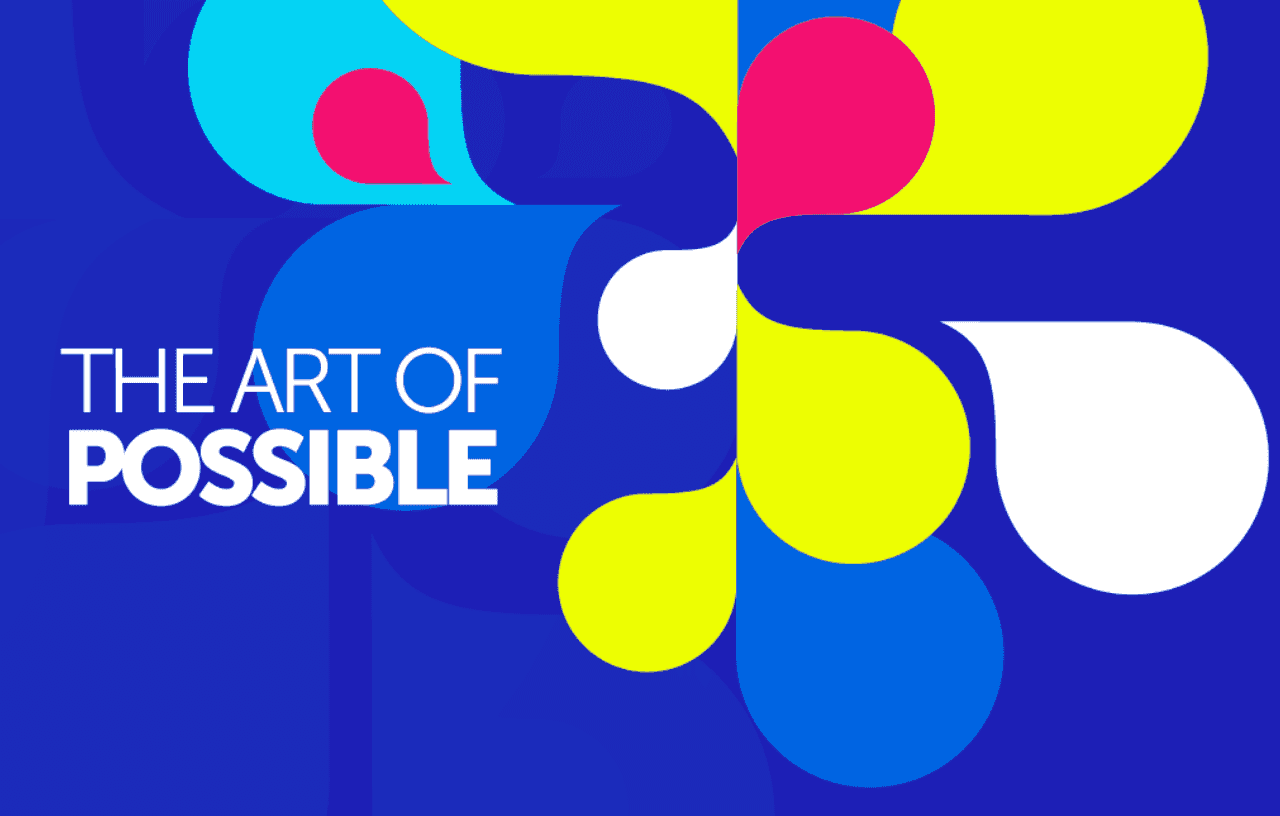
February 21, 2024
RSAC 2024: What’s New
SafeLiShare unveils groundbreaking AI-powered solutions: the AI Sandbox and Privacy Guard in RSAC 2024
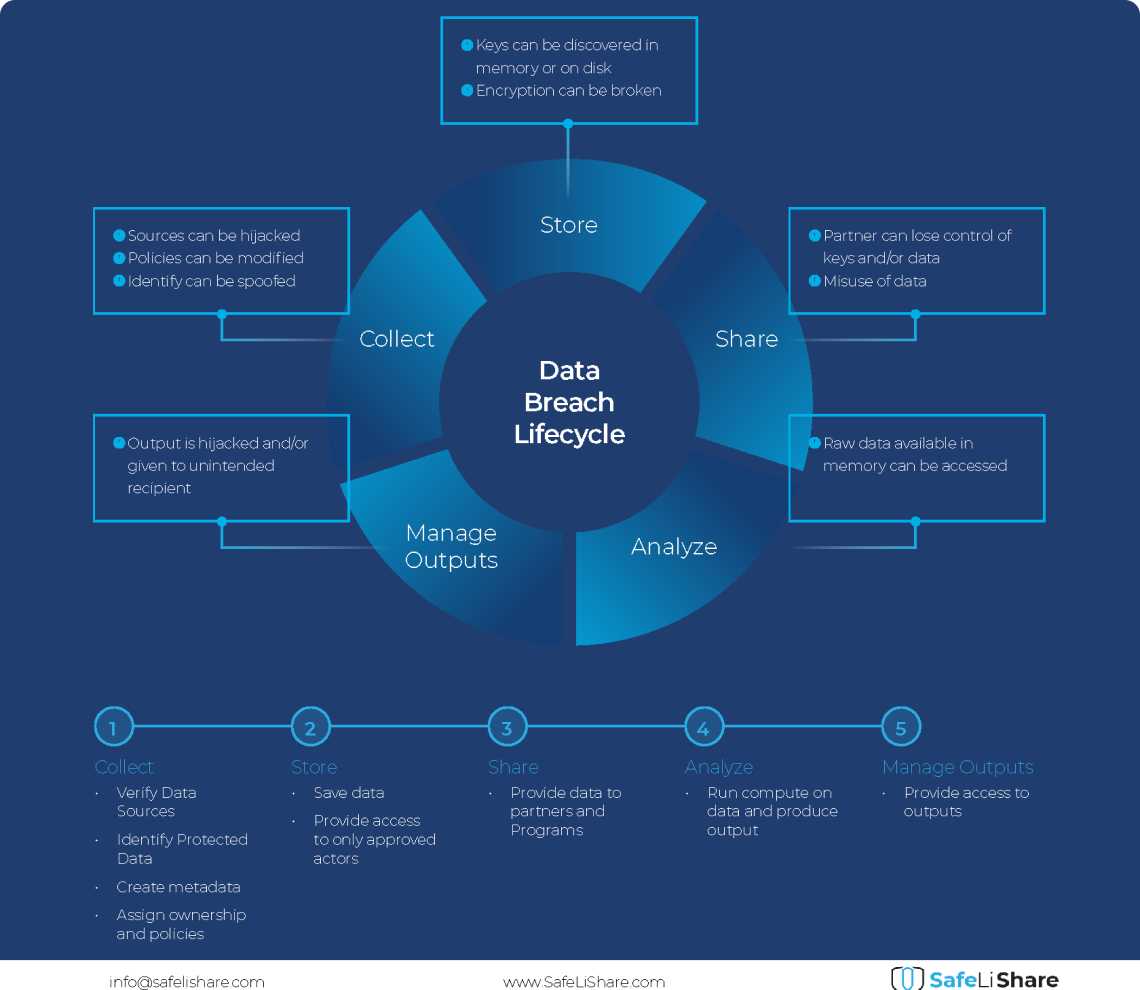
February 21, 2024
Cloud Data Breach Lifecycle Explained
During the data life cycle, sensitive information may be exposed to vulnerabilities in transfer, storage, and processing activities.
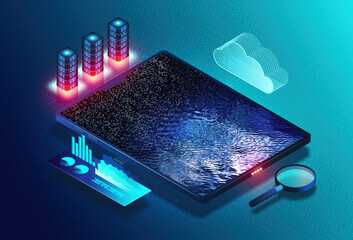
February 21, 2024
Bring Compute to Data
Predicting cloud data egress costs can be a daunting task, often leading to unexpected expenses post-collaboration and inference.
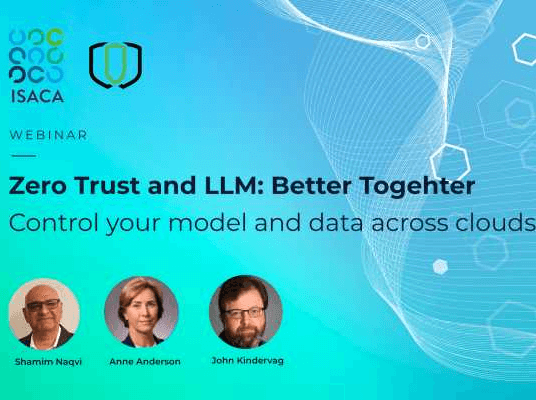
February 21, 2024
Zero Trust and LLM: Better Together
Cloud analytics inference and Zero Trust security principles are synergistic components that significantly enhance data-driven decision-making and cybersecurity resilience.