Zero Trust Principles in Generative AI
Delivers Run-Time Security for AI Models, Apps, and Agents
The use of hosted LLM and Generative AI models unlocks many benefits, but users must also contend with unique risks in three primary categories:
1. Content Anomaly Detection
- Unacceptable or Malicious Use: Zero Trust principles mandate continuous monitoring and verification to detect and prevent unacceptable or malicious use of AI models.
- Confidential Data Compromise: Enterprise content transmitted through prompts or other methods must be encrypted and monitored to prevent the compromise of confidential data inputs.
- Hallucinations and Inaccurate Outputs: Outputs must be scrutinized for accuracy and legality to avoid hallucinations, copyright infringement, and other unwanted results that could compromise enterprise decision-making.
2. Data Protection
- Data Leakage and Confidentiality: Zero Trust requires strict access controls and encryption to protect data in both hosted vendor environments and internal, self-managed environments.
- Governance of Privacy and Data Protection: Implementing Zero Trust helps enforce privacy and data protection policies, even in externally hosted environments.
- Privacy Impact Assessments: Zero Trust facilitates compliance with regional regulations by providing transparency and control over data handling, despite the “black box” nature of third-party models.
3. AI Application Security
- Adversarial Prompting Attacks: Zero Trust principles help mitigate adversarial prompting attacks by enforcing strict access controls and continuous monitoring.
- Vector Database Attacks: Secure enclaves and encryption protect against vector database attacks.
- Model State and Parameter Security: Zero Trust ensures that access to model states and parameters is tightly controlled and monitored to prevent unauthorized access.
A 2023 Gartner survey of over 700 webinar attendees validated these risk categories, highlighting data privacy as the top concern. These risks are exacerbated when using externally hosted LLM and other Generative AI models, as enterprises lack direct control over application processes and data handling. However, risks also exist in on-premises models, especially when security and risk controls are lacking.
SafeLiShare LLM Secure Data Proxy delivers Secure Enclave as a service with run-time encryption to address these risk categories. Responsibilities for implementing mitigating controls are split across two main parties, defined by their roles with regard to the AI models, applications, or agents.
Business Benefits (Use Cases)
Legacy controls are insufficient to mitigate risks associated with using hosted Generative AI models (e.g., LLMs). Users encounter risks in three shared responsibility categories. Entrepreneurial third-party vendors are addressing these vulnerabilities for three distinct use cases.
SafeLiShare LLM Secure Data Proxy
SafeLiShare’s Secure Enclave with LLM Secure Data Proxy (SDP) embodies the principle of “never trust, always verify” by integrating Open Policy Agent (OPA) with identity providers (iDP) and access control mechanisms through WAVE cache protection, while safeguarding LLM caches. This innovative technology provides full visibility and enforcement throughout the LLM Retrieval-Augmented Generation (RAG) workflow and addresses key risks in GenAI environments.
Input Risks: SafeLiShare mitigates the risks of input data compromise through content anomaly detection and filtering, ensuring that inputs such as API calls or interactive prompts comply with enterprise use policies. This protects sensitive information from being compromised by encrypting data in transit and at rest, while preventing inputs from violating legal and governance standards.
Output Risks: SafeLiShare also addresses the risks of unreliable or biased outputs from LLMs. Through anomaly detection and validation, it screens and filters outputs for copyright issues, hallucinations, and malicious content, safeguarding the enterprise from making misinformed decisions or violating intellectual property rights.
Data Protection: SafeLiShare ensures data confidentiality and governance, especially when interacting with external environments such as vector databases. It obfuscates sensitive data shared with hosted models and secures vulnerable integration points like API calls, protecting against breaches and unauthorized access.
Managing New GenAI Threat Vectors: The solution is designed to prevent adversarial prompting, prompt injection attacks, vector database breaches, and other security threats unique to AI applications.
Through collaboration with existing security providers like Security Service Edge (SSE), API Gateway or Data Loss Prevention (DLP), SafeLiShare provides additional layers of protection, filtering inputs and outputs to mitigate cybersecurity risks associated with third-party-hosted GenAI models.
Overall, SafeLiShare’s comprehensive approach combines OPA, WAVE, and LLM cache protection to secure data throughout the entire AI lifecycle, providing enterprises with confidence in the safety and compliance of their GenAI operations with Just in Time (JIT) security. This mechanism also works across the entire LLM chain, from data ingestion to prompt generation.
Experience Secure Collaborative Data Sharing Today.
Learn more about how SafeLiShare works
Suggested for you
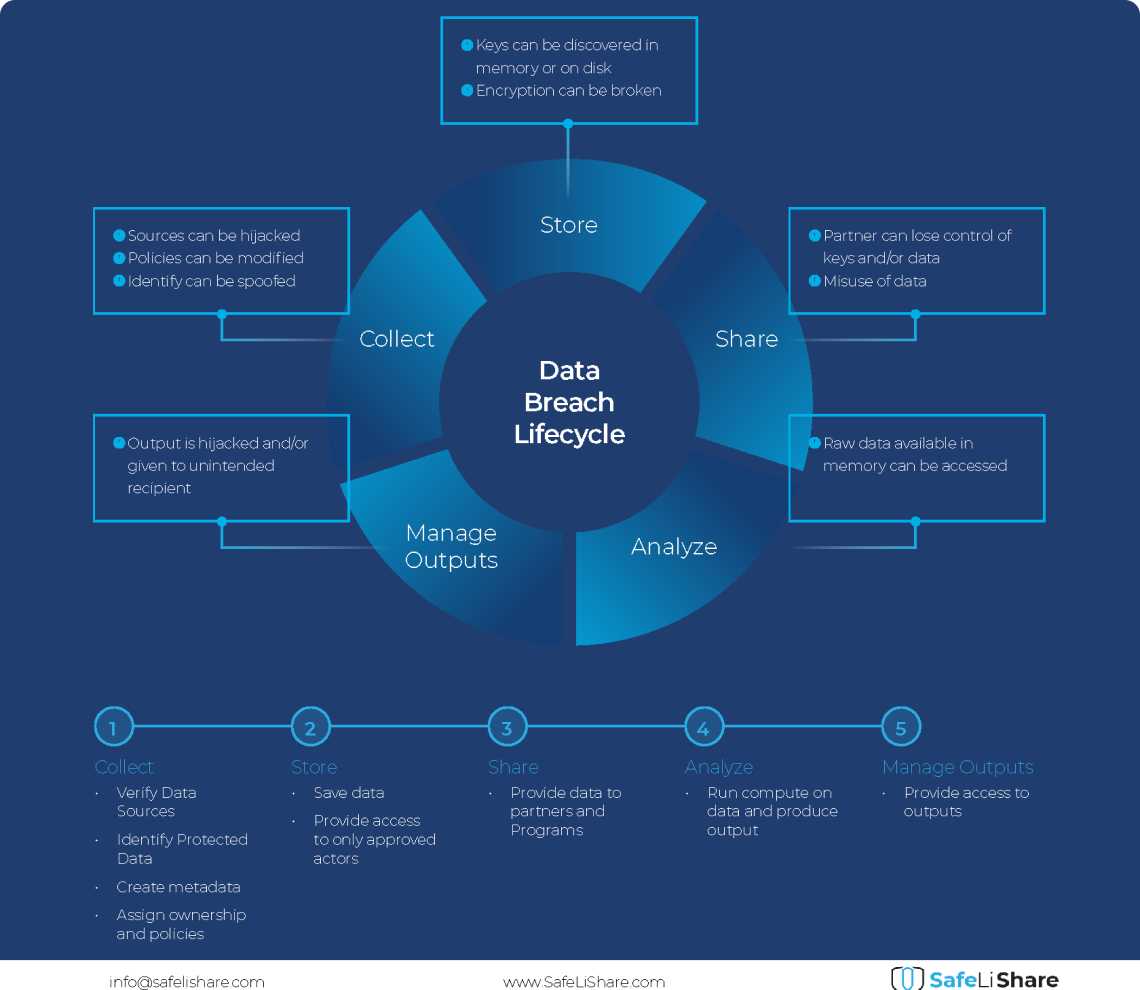
February 21, 2024
Cloud Data Breach Lifecycle Explained
During the data life cycle, sensitive information may be exposed to vulnerabilities in transfer, storage, and processing activities.
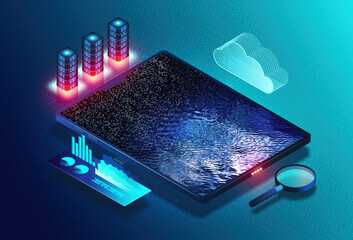
February 21, 2024
Bring Compute to Data
Predicting cloud data egress costs can be a daunting task, often leading to unexpected expenses post-collaboration and inference.
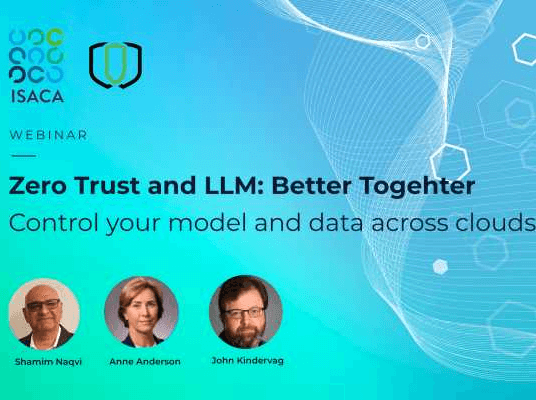
February 21, 2024
Zero Trust and LLM: Better Together
Cloud analytics inference and Zero Trust security principles are synergistic components that significantly enhance data-driven decision-making and cybersecurity resilience.