RSA 2023: Trends in Confidential Computing, AI, Data, Privacy
The annual RSA Conference(RSAC) #RSAC2023 was held last week in San Francisco, featuring many cybersecurity professionals aiming to attract customers, prospects, and partners. The news cycle following the conference is expected to last for some time. Here are my impressions of the most compelling aspects of the event.
This year’s RSAC features artificial intelligence(AI) as a prominent topic, while zero trust is not as emphasized.
The theme chosen for AI this year focused on generative AI, particularly Chat GPT, which has received significant attention in recent times.
Applying AI to cybersecurity is as fascinating as the challenges it poses in terms of literary license and intellectual property rights. According to Jeetu Patel, Executive Vice President and General Manager of Security and Collaboration of Cisco, the cybersecurity industry is currently at an “inflection point” with AI and cybersecurity. Several solution vendors leverage AI to support security operation centers (SOC), while others focus on securing AI. It may be possible to secure data from AI bots or application training by limiting designated applications and data linkage to process and train data with zero trust or machine-scale root of trust.
Note that the model, the data, and the experience must come together to make AI generate improved insights. That makes data clean room, application identity, and access control over data assets technologies critical.
Data loss prevention (DLP) and data access control are well-known things. How do we harden AI and machine learning (ML) modeling, and training with privacy-enforced privilege? How do you achieve privacy preservation at the cloud and machine scale? One thing that’s commonly missing in these approaches is an attempt to harden access control from within by hardening the entity trying to access the data.
Confidential Computing is Cloud Computing with Privacy and Secret Enforcement
Some of the recent trends and news from RSA 2023 include the introduction of Confidential Computing VM instances that utilize AMD Infinity Guard Technology, specifically AMD SEV-SNP offering new hardware-based security protections covering memory protection and attestation. Some reports also cover news about Intel’s upcoming confidential computing technology Trusted Domain Extensions (TDX).
Google’s recently announced Confidential Spaces enables various privacy-focused computations such as joint data analysis on joint datasets, and machine learning model training with trust guarantees that the data stays private and not observable by cloud providers. Confidential computing-based sandboxes/clean rooms will allow the phasing out of third-party cookies and limit covert tracking, thus providing ad techs safer alternatives to engage in their business while keeping user data private.
Security Consolidation is Here
Securing AI in training data
Securing AI in training data involves ensuring that the data used to train the AI model is accurate, unbiased, and free from any malicious intent. This can be achieved through various methods such as data validation, data cleansing, and data encryption. Data validation involves checking the data for errors or inconsistencies, while data cleansing involves removing any irrelevant or duplicate data. Data encryption involves protecting the data from unauthorized access or tampering. By securing the training data, we can ensure that the AI model is reliable and produces accurate results.
Another trend related to confidential sandboxes and clean rooms is that of cloud-based caches and data storage areas & data vaults protected by confidential computing technology. In such storage areas, enterprise data stored in datasets, buckets, databases, data lakes, and streaming data become data sources from which specified data is extracted. This allows existing enterprise data to be protected against data loss since applications may now use the extracted data without having the need to access the original enterprise data sources. The extracted data is then treated as an asset, e.g., it may be encrypted for protection using envelope encryption where the encryption keys are managed by Trusted Execution Environment technology. Such assets may then be made available under policy control to applications executing in confidential computing-controlled environments. Additional security considerations may entail the use of hardware-based application identity-controlled access mechanisms and hardware-based root of trust attestation.
Airtight your data privacy, cloud data privacy, prevent data loss
SafeLiShare creates a mesh to help organizations with their trust boundaries with full asset-based integrity, transparency, and access control. We provide a confidentiality enforcement wrapper, whereby you don’t need to know that the confidential compute layer exists. Whether you have data in:
- public data (data that can reside in public clouds)
- restricted data (formerly unable to move to the public cloud, now able to be moved to the public cloud if confidentiality can be guaranteed)
- critical data (cannot move to the public cloud).
The main battlegrounds right now are moving sensitive/private workloads to the cloud with
- cloud-native apps (that have to process data that isn’t all on the cloud)
- data lakes (overwhelming amount of data)
To ensure the security of AI, it is important to prioritize the protection of classified data with sensitivity and confidentiality measures.
Summary
- Data Loss Prevention is already well known and mature: data is being accessed by more than just humans now, it’s being accessed by applications as well.
- Everybody has a data security solution already, no matter what it is. AI security should be what new data security companies are primed for. Machine scale needs data security from the inside out.
- Internalize zero trust initiative and principles from the hardware-enforced application — data linkage with automated “always verify, never trust” ledger and tamper-proof audit logs for downstream threat behavior processing.
- The time-consuming homomorphic encryption approach is slow and costly. Innovate current commodity encryption technology i.e. PKI for uni-party or multiparty real-time data collaboration and AI ML model training.
- Data in general is destined/used for AI.
- Overarching security consolidation and automation at the machine-scale and cloud-scale are here to avoid silos, tool sprawls, and human configuration errors. Know what ecosystem partners you are paired with and who you sell with and what solutions you are including.
For more information about SafeLiShare’s Encrypted Data Clean Room, Federated Learning, and how you can abstract the cloud-agnostic data protection across Azure, AWS, and GCP, schedule a demo to see it in action or click here.
Definition:
Confidential Computing
Confidential computing is Cloud Computing. It refers to a set of next-gen security technologies and practices that aim to protect sensitive data and processes from unauthorized access, disclosure, and manipulation. The key idea behind confidential computing is to create a secure and isolated environment, often called a trusted execution environment (TEE), where data and applications can be processed without the risk of exposure to external threats or malicious actors. This is achieved through a combination of hardware and software-based security measures, such as encryption, secure boot, memory isolation, and attestation, which ensure that the data and processes remain confidential and trustworthy even in the presence of potential attacks. Confidential computing has many applications in areas such as cloud computing, data protection, and privacy-sensitive industries, where the need for secure and private processing of sensitive data is paramount. It’s available in AWS, Azure, GCP, and IBM, etc. Visit SafeLiShare.com for more use case introductions.
Suggested for you
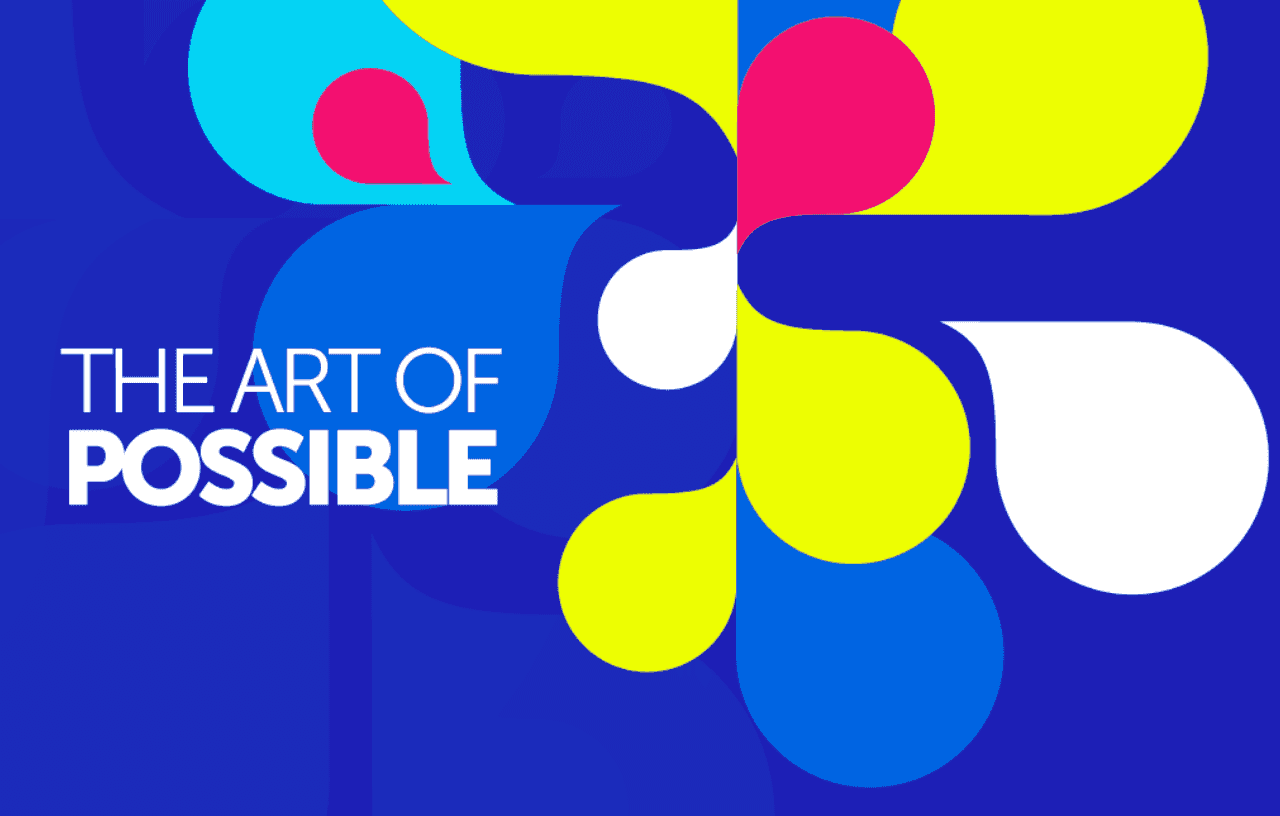
February 21, 2024
RSAC 2024: What’s New
SafeLiShare unveils groundbreaking AI-powered solutions: the AI Sandbox and Privacy Guard in RSAC 2024
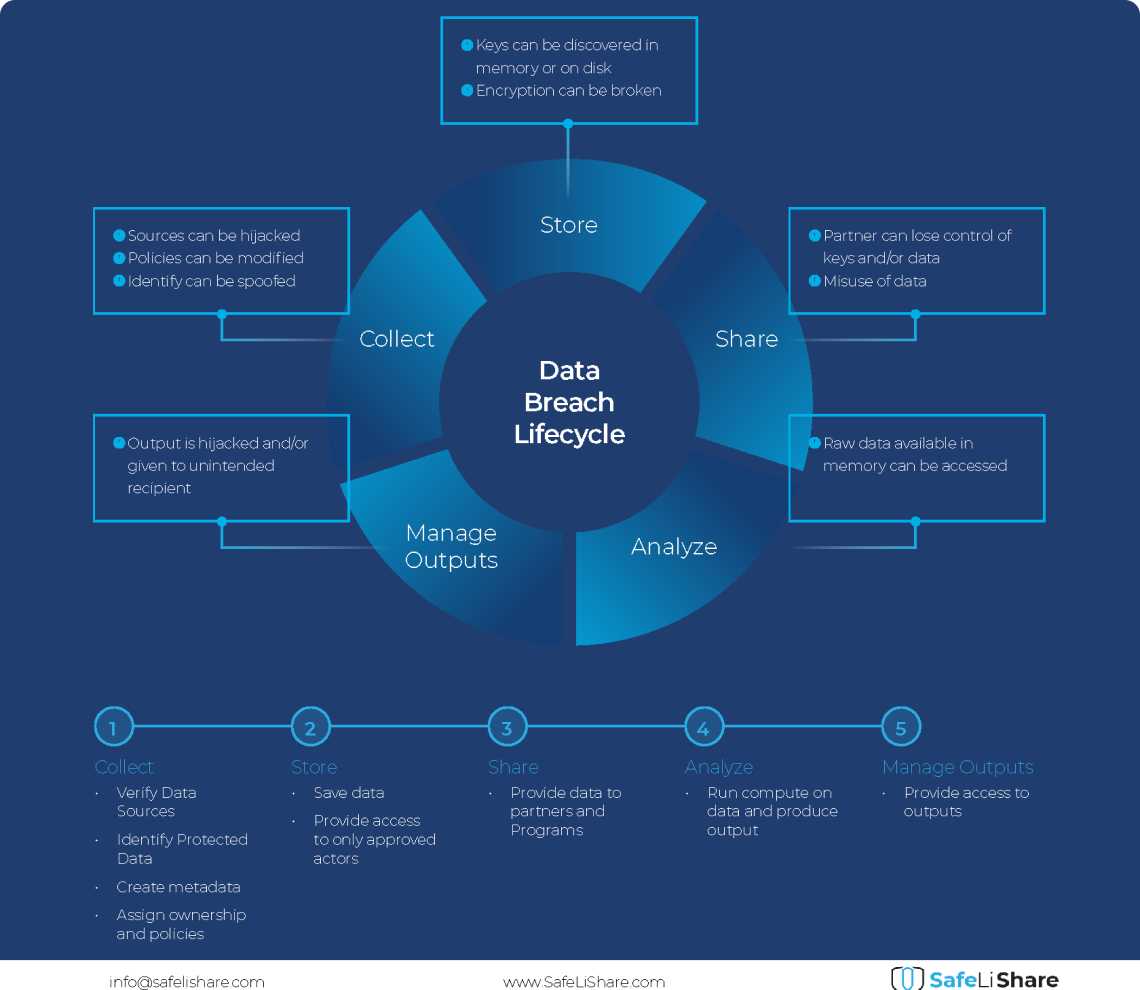
February 21, 2024
Cloud Data Breach Lifecycle Explained
During the data life cycle, sensitive information may be exposed to vulnerabilities in transfer, storage, and processing activities.
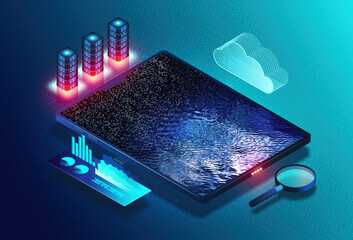
February 21, 2024
Bring Compute to Data
Predicting cloud data egress costs can be a daunting task, often leading to unexpected expenses post-collaboration and inference.
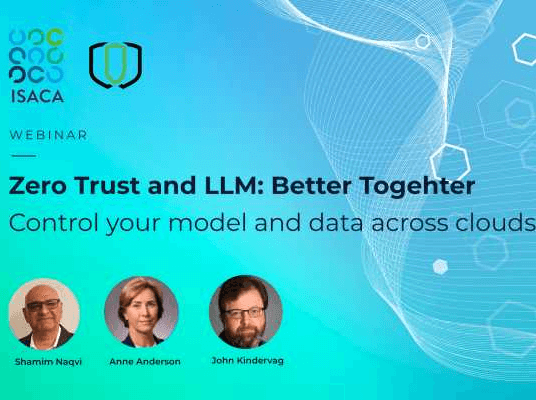
February 21, 2024
Zero Trust and LLM: Better Together
Cloud analytics inference and Zero Trust security principles are synergistic components that significantly enhance data-driven decision-making and cybersecurity resilience.